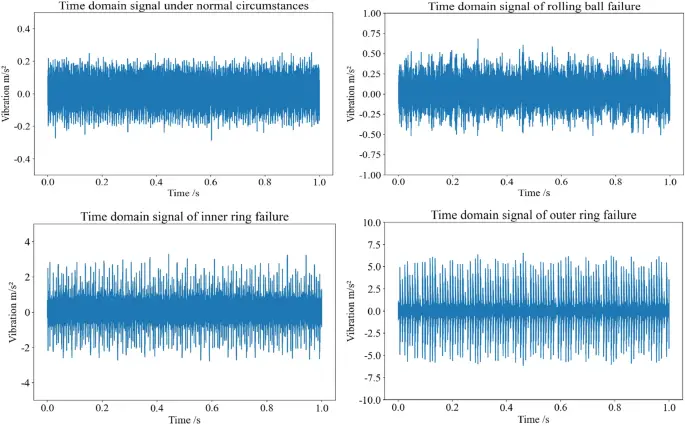
Revolutionizing Bearing Fault Detection: Harnessing the Potential of Genetic Algorithms and Neural Networks
Key Takeaways:
Bearing faults can have severe consequences on machinery performance, leading to unexpected breakdowns, increased downtime, and potential safety hazards. Hence, it is crucial for industries to invest in effective fault detection methods to maintain the integrity and reliability of their machinery. In recent years, the integration of genetic algorithms and neural networks has revolutionized bearing fault detection, enabling engineers to create advanced predictive maintenance systems. This article explores the power of genetic algorithms and neural networks and their potential to revolutionize the field of bearing fault detection.
Understanding Bearing Faults
Bearing faults occur due to various factors such as material fatigue, contamination, improper lubrication, and excessive loads. These faults often manifest as abnormal vibrations, unusual sounds, or unpredictable temperature variations. Detecting these faults at an early stage is crucial to prevent further damage and mitigate the risk of catastrophic failures.
Historically, fault detection methods relied on traditional statistical approaches and rule-based algorithms. While these methods were effective to some extent, they lacked the ability to adapt to complex and dynamic machinery conditions. This limitation led to the development of artificial intelligence techniques, particularly genetic algorithms and neural networks.
The Power of Genetic Algorithms in Fault Detection
Genetic algorithms (GAs) were inspired by the process of natural evolution and Darwin’s theory of survival of the fittest. They are a computational approach to solving optimization problems by mimicking the principles of genetics and natural selection. GAs maintain a population of potential solutions, apply genetic operators such as mutation and crossover, and evaluate their performance based on a fitness function. Over multiple generations, the algorithm refines the solutions, converging towards an optimal or near-optimal solution.
In the context of bearing fault detection, genetic algorithms can be employed to optimize feature selection, allowing the system to identify the most relevant features from vast datasets. By selecting the most discriminative features, GAs enhance the accuracy and efficiency of fault detection algorithms. Ultimately, this leads to more reliable predictions and reduces false alarms or missed detections.
Unleashing the Potential of Neural Networks
Neural networks are computational models inspired by the human brain’s ability to learn and recognize patterns. These networks consist of interconnected nodes, or artificial neurons, organized in layers. Each neuron takes in input, processes it, and produces an output using activation functions.
In bearing fault detection, neural networks are trained using historical data, particularly labeled datasets containing examples of faulty and non-faulty bearing conditions. The network learns the complex relationships between input features and corresponding fault types, enabling it to identify faults in unseen datasets.
The synergy between genetic algorithms and neural networks is particularly powerful in this domain. Genetic algorithms optimize the neural network’s structure and parameters, improving its performance and generalization ability. By intelligently tuning the network’s architecture and weights, the system becomes highly adaptive and robust, capable of handling various operating conditions and machine configurations.
Applications Beyond Bearing Fault Detection
Although the primary focus of this article is on the promising potential of genetic algorithms and neural networks in bearing fault detection, it is essential to highlight their broader applications. The power of these techniques extends beyond fault detection and encompasses various areas, including but not limited to:
- Image recognition and computer vision
- Natural language processing and sentiment analysis
- Finance and stock market prediction
- Healthcare and medical diagnostics
These versatile tools offer significant advantages in terms of accurate predictions, efficiency, and scalability. As the world becomes increasingly digitized, the application of genetic algorithms and neural networks will continue to grow across multiple industries.
Frequently Asked Questions
[//myfaq]
Conclusion
In conclusion, the amalgamation of genetic algorithms and neural networks presents an exciting opportunity to revolutionize bearing fault detection. These artificial intelligence techniques offer unparalleled accuracy and efficiency, minimizing downtime, reducing maintenance costs, and maximizing machine reliability.
By harnessing the power of these revolutionary approaches, industries can proactively predict and prevent bearing faults, ensuring the longevity and performance of their machinery. As technology advances and datasets grow, genetic algorithms and neural networks will continue to lead the way in predictive maintenance, transforming industries worldwide.
With promising applications extending beyond bearing fault detection, the future of genetic algorithms and neural networks is bright. Embracing and harnessing these powerful tools will unleash unprecedented potential, propelling industries into a new era of innovation and efficiency.
Source: insidertechno.com