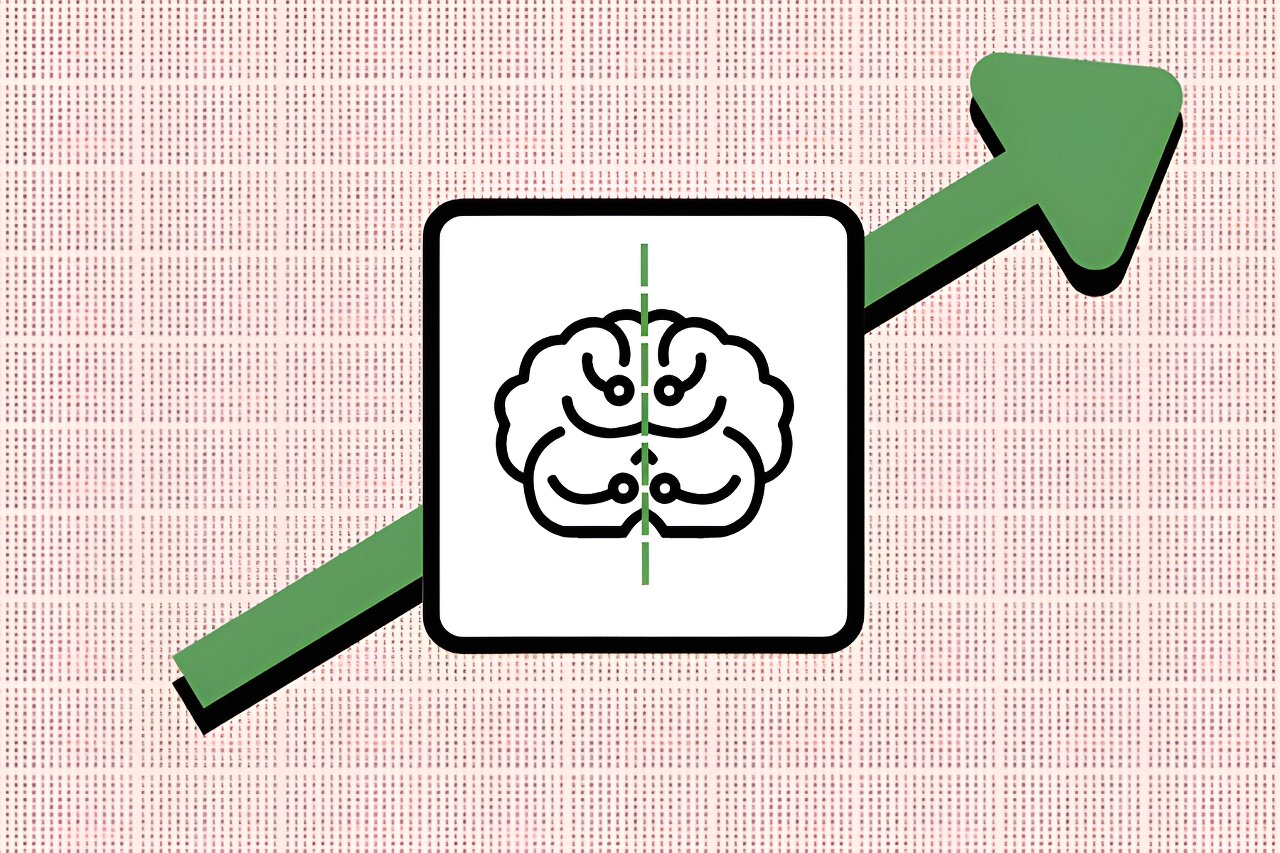
Key Takeaways:
Unveiling the Secrets: How Symmetry Enhances Machine Learning
The world of machine learning is increasingly dominated by complex algorithms and models. To truly harness the power of machine learning, it is essential to understand the role that symmetry plays in enhancing its performance. By seamlessly integrating the concept of symmetry, we can unlock the hidden potential and achieve greater accuracy and efficiency in our machine learning models. In this article, we will delve into the secrets of how symmetry enhances machine learning.
The Importance of Symmetry in Machine Learning
Symmetry can be a powerful tool in improving the effectiveness of machine learning algorithms. It provides a structured framework for organizing and understanding complex data patterns, allowing machine learning models to make more accurate predictions.
One key area where symmetry shines is in data preprocessing. By identifying and exploiting symmetries in the data, we can greatly reduce the amount of computation required to process it. This leads to faster model training and inference, ultimately saving time and computational resources.
In addition to efficiency gains, symmetry also enhances generalization. Machine learning models that learn from symmetric or partially symmetric data tend to be more robust and less prone to overfitting. This is because the symmetry encodes certain structural characteristics of the data, leading to improved performance on unseen examples.
The Role of Symmetry in Model Design
Symmetry is not limited to the data itself; it also plays a crucial role in the design of machine learning models. By incorporating symmetries into the model architecture, we can achieve better performance and interpretability.
One well-known example is convolutional neural networks (CNNs). CNNs exploit the translational symmetry present in images to effectively detect and extract features at different spatial locations. This leads to superior performance in tasks such as image classification, object detection, and segmentation.
In addition to translational symmetry, other types of symmetries can also be leveraged in model design. For example, symmetries in the relationship between different input features can be incorporated through techniques such as weight sharing or group equivariant layers. These approaches enable the model to effectively learn from the symmetries present in the data, enhancing its learning capacity.
The Impact of Symmetry in Training Techniques
Symmetry is not only relevant in the design and preprocessing stages of machine learning. It can also significantly impact the training techniques used to optimize model performance.
One important aspect to consider is the regularization of models. Regularization techniques, such as weight decay or dropout, can be tailored to leverage the underlying symmetry in the data. This not only improves model generalization but also prevents the model from being biased towards particular symmetries, leading to more robust and unbiased predictions.
Furthermore, symmetries can also be directly incorporated into optimization algorithms. For example, certain optimization algorithms, such as group-lasso or proximal operators, are specifically designed to handle structured and symmetric models. These algorithms exploit the underlying symmetry in the model and can greatly improve both training efficiency and convergence speed.
Incorporating Symmetry into Machine Learning Workflows
Now that we understand the importance of symmetry in machine learning, it is essential to know how to incorporate it into our existing workflows.
First and foremost, recognizing the presence of symmetry in the data is crucial. This can be achieved through visual inspection, data exploration, or domain knowledge. Once identified, appropriate techniques, such as data augmentation or symmetry-based model architectures, can be applied to take advantage of the symmetry.
Additionally, staying up-to-date with the latest research and advancements in symmetry-aware machine learning can provide valuable insights and techniques to further enhance our models.
Frequently Asked Questions
Conclusion
Symmetry plays a crucial role in enhancing machine learning techniques. By leveraging the power of symmetry, we can improve the efficiency, accuracy, and robustness of our machine learning models. Whether it is through data preprocessing, model design, or training techniques, symmetry has the potential to unlock the full secrets of machine learning. Embracing symmetry-aware approaches will undoubtedly pave the way for exciting advancements in the field of machine learning.
Source: insidertechno.com